Increasing numbers of companies are now looking to Artificial Intelligence (AI) to make them work better, and the figures speak for themselves. As per Forbes Advisor, 72% of companies are currently employing AI for one or more business operations, and nearly half are utilizing it in several areas.
From responding to customer questions quickly to improving decision-making and automating routine tasks, AI is assisting businesses in saving time, reducing expenses, and remaining competitive. However, simply leveraging AI tools is not sufficient. Businesses must incorporate AI into their daily operations in a strategic and intentional manner to truly experience the benefits.
In this blog, we’ll explore why AI integration matters, how to do it right, and share a real success story to show what’s possible when it’s done well.
Importance Of Integrating AI In Business
As AI continues to evolve, its value in the business world is becoming undeniable. Whether you’re a startup looking to scale efficiently or an enterprise aiming to maintain a competitive edge, integrating AI is no longer optional—it’s strategic. AI is empowering businesses to think smarter, act faster, and operate seamlessly. Let’s take a look at its importance:
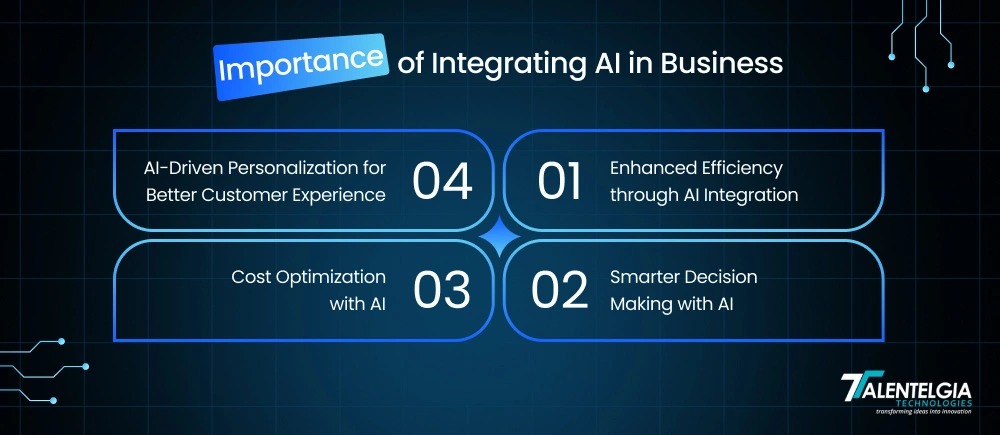
1. Enhanced Efficiency
AI isn’t automation—it’s elevation. By automating tedious and time-consuming tasks such as data entry, appointment booking, customer service, and even reporting, AI enables teams to reallocate their efforts to more significant, strategic work. This unleashes greater productivity, creativity, and innovation within departments.
McKinsey says that AI and business automation technologies can process 60%–70% of mundane tasks, largely eliminating operational workload and enhancing the productivity of employees. This transition enables workers to focus on critical thinking, problem-solving, and value-based decision-making.
Case Study: Reclaim.AI
Reclaim.ai is a productivity tool powered by AI that optimizes individuals’ and teams’ schedules by defending focus time, managing tasks, and eliminating unnecessary meetings automatically.
Impact Snapshot:
- 395 hours of focus time/user/year
- 7.6 more productive hours/week
- 2.3 fewer unnecessary meetings/week
- 3.8 hours/week saved managing time
Utilized by more than 500,000 professionals working in 60,000+ companies, Reclaim enables teams to focus on deep work, automate scheduling meetings, and create healthier habits using AI. With goal-setting for Focus Time and smart scheduling, users claim up to 40% more time reclaimed, without overtime work.
Use Case: Suitable for remote teams, managers, and freelancers balancing conflicting priorities, Reclaim improves performance without burnout.
2. Smarter Decision Making
AI has changed the decision-making for businesses by delivering rich, data-backed insights. No longer do decision-makers need to resort to guesswork or tedious analysis; AI systems can sift through huge sets of data, identify concealed patterns, and recommend the optimal solution, quickly than any human.
And business leaders are listening. A Deloitte poll showed that almost 6 out of 10 executives reported that AI enabled them to derive more actionable insights from their data.
But in which industries and how AI optimizes decision-making varies:
- In the energy sector, Shell employs predictive analytics with AI to identify where best to drill and predict the equipment maintenance needed, reducing downtime and making better resource usage.
- In healthcare, Watson at IBM draws from clinical documents and medical research to assist doctors with selecting the best, evidence-driven cancer therapies tailored to an individual patient’s characteristics.
Regardless of your industry—retail, logistics, finance, or otherwise—AI enables you to make better, quicker decisions with confidence by:
- Converting raw data into actionable insights
- Identifying patterns that humans tend to overlook
- Predicting future trends and risks
- Facilitating real-time decisions based on up-to-the-minute market conditions
In an environment where customer behavior shifts overnight and market trends shift quickly, AI gives business leaders the clarity, speed, and precision they require to stay ahead.
Case Study: IBM Watson
IBM Watson® Studio is a powerful AI and machine learning platform that enables teams to develop, train, and deploy AI models quickly. It is built to facilitate smarter, data-informed decisions across businesses and industries by bringing together developers, data scientists, and analysts on one unified collaboration platform.
Impact Areas:
- MLOps: Watson Studio automates model AI development and deployment through automated machine learning and lifecycle management capabilities.
- Decision Optimization: Business users can rapidly create dashboards and execute optimization models to decide the optimal action in sophisticated situations.
- Visual Modeling & NLP: Users can conduct high-quality text analysis and visualize data naturally with SPSS-style workflows and pre-trained NLP models in more than 20 languages.
- AutoAI: This capability automates data prep, model development, and tuning, allowing both new and experienced developers to speed up development and experimentation.
Business Value Delivered:
- Decreased model monitoring activity by 35%–50%
- Increased model accuracy by 15%–30 %
- Facilitated deployment of AI across multicloud environments through REST APIs
- Increased collaboration and lowered operational expenses by aligning tools and teams
With IBM Cloud Pak® for Data, Watson Studio brings multicloud AI to reality—enabling businesses to optimize decisions, ensure compliance, and extend AI across the enterprise.
3. Cost Optimization
One of the strongest business cases for AI adoption is cost reduction, and with good reason. AI applications excel at repetitive task automation, freeing teams to perform higher-level and creative work. This not only improves productivity but also improves morale and retention rates.
This is how AI makes businesses reduce their costs:
- Predictive maintenance: AI is able to forecast when machinery will fail, enabling companies to maintain equipment ahead of time, preventing costly breakdowns.
- Optimal supply chains: AI takes demand patterns and delivery information to minimize inventory losses and accelerate logistics, making the operation more streamlined.
- Energy optimization: AI systems enable companies to monitor and maximize their energy consumption through analyses of heating, cooling, and lighting patterns. Not only does this lower utility bills, but it also reduces carbon emissions to a substantial degree.
Given that construction operations by themselves drive 30% of the world’s final energy use and 26% of the world’s energy-related emissions (International Energy Agency), the difference that smarter energy management makes is gigantic. AI is also featured by the World Economic Forum as a climate solution, and they put the impact at reducing 5–10% of global greenhouse gas emissions by 2030. Not surprisingly, therefore, the IEA considers AI “a tool in cutting emissions”—and a smart tool to boot.
Case Study: How Google Used DeepMind AI to Cut Data Center Cooling Energy by 40%
Over the last decade, energy efficiency has been a core area of interest for Google. From designing special energy-efficient servers to embracing pioneering green energy measures, the company has repeatedly reset the way large-scale infrastructure can be made sustainable. But the most revolutionary shift came with the inclusion of DeepMind’s artificial intelligence in its data center operations.
Let’s dissect how this strategic action has become a gold standard in energy optimization among businesses globally.
The Problem: Enormous Energy Consumption in Data Centers
Data centers are the virtual engines powering searches such as Google Search, Gmail, and YouTube. However, these operations produce massive heat, prompting the need for intricate cooling systems to avoid overheating.
This cooling is usually done with pumps, chillers, and cooling towers—all of which require high amounts of energy. But dynamically managing cooling is difficult because:
- Environmental conditions are constantly in flux (such as weather)
- Sophisticated interactions between architecture and equipment
- Lack of rule-based engineering and human insight
Every data center is uniquely configured, so it is practically impossible to have a one-size-fits-all solution.
The Solution: DeepMind AI for Smart Cooling
Google started working with DeepMind—its artificial intelligence research unit—to create a machine-learning-based control system that could manage these intricate variables more smartly than conventional approaches.
Here’s what they did:
- Data Collection: Google drew on massive streams of historical data from thousands of sensors across its data centers, tracking factors such as temperature, power usage, humidity, and pump speeds.
- AI Modeling: DeepMind used this data to train deep neural networks to forecast energy consumption patterns and compute optimal cooling plans in real time.
- Smart Forecasting: The AI was taught to model how various cooling adjustments would affect important metrics such as temperature, pressure, and Power Usage Effectiveness (PUE).
The Results: Up to 40% Less Cooling Energy
DeepMind’s AI models were tested in real-world environments with astounding results:
- 40% reduction in cooling energy consumption
- 15% lower total energy overhead (PUE)
- The lowest recorded PUE for that specific site
These efficiencies are even more compelling given the way Google’s data centers were already optimized. It’s an eloquent showing of how AI is able to realize latent efficiencies in even finely calibrated systems.
4. Personalization
In today’s experience-driven economy, customer expectations have evolved. Shoppers now want interactions that feel personal, timely, and genuinely helpful, not generic. This is where AI is stepping in as a game-changer, reshaping how businesses connect with their audiences.
Modern AI systems can process vast amounts of customer data—browsing patterns, purchase history, real-time behaviors, and even emotional cues—to deliver experiences that feel tailor-made for each user. From personalized product suggestions to intelligent support that anticipates a customer’s needs, AI helps create a sense of one-on-one service at scale.
But it doesn’t stop there.
AI-driven personalization enhances:
- Product discovery, by recommending items aligned with individual preferences.
- Customer engagement, by creating dynamic website content or email campaigns that resonate.
- Support experiences through chatbots that adapt responses based on user history and tone.
- Predictive insights help brands understand what customers are likely to want next, even before they ask for it.
This blend of personal relevance and immediacy not only improves satisfaction but also drives loyalty and repeat business. Research shows that personalization can boost conversion rates by over 40% while also significantly reducing churn.
Case Study: Nosto
Nosto offers an effective, AI-powered platform to transform your e-commerce site into a personalized, high-converting shopping experience. Use rich commerce insights and deep machine learning, and Nosto helps brands deliver conversion and customer-retention experiences.
With Nosto’s experience, AI™, you can:
- Construct Dynamic Personalization: Use data-driven insights to offer personalized product recommendations, enhance search results, and construct individualized merchandising displays.
- Optimize Performance through Automation: Nosto’s technology automates targeted campaign suggestions for optimal performance and operational efficiency, with minimal effort required.
- Harmonize Your Commerce Data: With real-time analytics and easy-to-use dashboards, Nosto brings together your customer data, providing open visibility for improved decision-making and channel performance measurement.
By incorporating Nosto’s AI-driven personalization into your eCommerce strategy, you can boost customer satisfaction, accelerate revenue growth quickly, and create memorable shopping experiences that keep your brand top-of-mind.
7 Steps To Integrate AI In Business
Integrating AI into business isn’t just about keeping up—it’s about staying ahead. With proven benefits across productivity, decision-making, cost-efficiency, and personalization, AI is becoming essential for long-term growth and competitiveness. Let’s take a closer look at the steps:
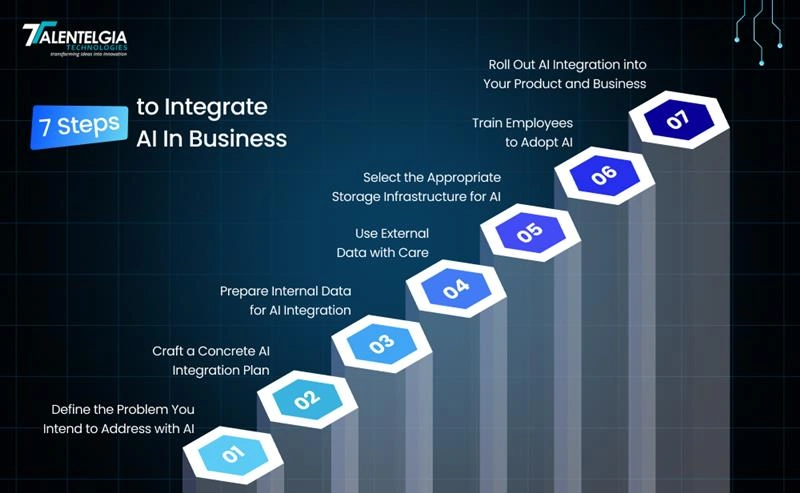
Step 1: Define the Problem You Intend to Address with AI
Before embarking on AI deployment, it is crucial to define the exact business problem that AI will address. AI isn’t being adopted to chase novelty but to address actual pain points. Some common uses for AI include:
- Personalizing customer interactions (such as Netflix’s AI-based recommendation engine)
- Streamlining supply chain operations (such as Walmart leveraging AI for inventory management and demand prediction)
- Enhancing cybersecurity (e.g., Darktrace’s AI-driven threat detection system)
- Enhancing inventory management and forecasting (e.g., Amazon leveraging AI for real-time inventory monitoring)
- Streamlining repetitive, data-intensive processes (e.g., UiPath’s robot process automation for management of repetitive manual processes)
Having a clear idea of what the problem is will assist in identifying what forms of AI technologies and sources of data are required so the integration yields concrete operational and financial advantages.
Step 2: Craft a Concrete AI Integration Plan
An AI strategy is instrumental in directing your integration process. It must outline:
- Integration goals and objectives
- Technologies required
- Data sources to be harnessed
- Monitoring and performance measurement metrics
- Change management plan
For instance, when Tesla brought out its autonomous vehicles, the company developed an overall strategy centered on vehicle autonomy improvement, real-time data collection from drivers, and using machine learning for better prediction. Tesla’s AI strategy remains dynamic based on user data, safety standards, and market requirements.
While this plan will change over time as your AI adoption reaches maturity, establishing a starting roadmap is important for clarity, alignment, and focus. Designating an AI integration leader or committee can also facilitate this effort.
Step 3: Prepare Internal Data for AI Integration
AI performance is highly reliant on quality data. Optimize your internal data assets before investing in new data infrastructure. Actions to ensure data readiness involve:
- Gathering isolated data sources to aggregate data lakes (e.g., Spotify aggregates user behavior and listening history into a single platform to make tailored music suggestions).
- Data cleansing and consistent data formatting are regularly maintained (e.g., Google maintains consistent data formats on different systems to build proper machine learning models).
- Developing internal data exchanges for allowing transparent data exchange among systems in real time (e.g., a data exchange on which Netflix builds so that recommendation algorithms stay constantly updated).
- Implementing data governance practices to maintain data security, access rights, and continuous quality assurance (e.g., IBM Watson maintains strict data governance for the healthcare industry to preserve patient confidentiality).
By keeping your internal data organized and accessible, you set the stage for successful AI implementation.
Step 4 : Use External Data with Care
If your internal data is lacking, consider ethically sourced external data. Third-party data can fill gaps, but be sure to:
- Confirm usage rights for external data sources
- Maintain consistency in data formatting with internal standards
- Screen external datasets from reputable vendors, like open-source databases or licensed market research (e.g., Weather.com provides weather data that businesses utilize for predictive models)
For example, Zillow incorporates outside real estate market data to complement its property value estimation models to make it more accurate.
Read More: Zillow API Cost
Incorporating outside data must be consistent with your internal data governance practices to ensure consistency and security.
Step 5: Select the Appropriate Storage Infrastructure for AI
AI integration requires strong data storage systems that can manage large volumes of data and offer efficient access to AI models. Some of the options are:
- Data lakes to store raw, unstructured data (e.g., LinkedIn utilizes a data lake to manage varied types of data, such as user activity, job postings, and engagement metrics)
- Data warehouses to perform analytical processing of structured data and business intelligence (example: Salesforce employs a data warehouse to study customer interactions and sales statistics)
- Data marts to perform specialized analytics specific to specific departments (example: Target employs data marts to offer real-time data to its marketing teams)
- Hybrid cloud implementations that integrate on-premise and cloud storage capabilities (example: Microsoft Azure provides hybrid solutions for companies that incorporate AI models)
In choosing storage infrastructure, keep in mind the factors of cost, scalability, compatibility of tools, and data security for hassle-free AI integration.
Step 6: Train Employees to Adopt AI
AI adoption works best when it empowers employees. Prioritize training your employees to learn to adapt to the changes brought about by AI, such as:
- Upskilling for new roles and responsibilities as AI automates mundane tasks (e.g., Boeing offers training for its engineers to work with AI in aircraft design)
- Educating teams to decode AI-driven insights in making good business decisions (e.g., how Coca-Cola uses AI to improve marketing campaigns and requires marketers to decode data-driven insights)
- Providing ongoing skill enhancement for employees to shift to more strategic job functions (e.g., Accenture has introduced upskilling programs for employees shifting to AI-based jobs)
A trained, flexible workforce will be key to success in integrating AI and making workers feel comfortable using AI.
Step 7: Roll Out AI Integration into Your Product And Business
Having done all this, start rolling out AI into your systems. Do this in stages:
- Pilot testing with small data sets and user bases to optimize AI models (e.g., Uber conducted pilot tests of its autonomous vehicles to realize safe and effective integration)
- Gradual integration within core systems to reduce risks (e.g., IBM Watson Health incrementally integrated AI into healthcare systems with analysis of clinical trial data)
- Ongoing monitoring of critical metrics like model accuracy, performance, and user experience (e.g., Facebook regularly updates its AI models using user engagement metrics)
A phased integration strategy enables controlled experimentation, allowing your team to learn from early deployments and refine AI capabilities as you grow.
Conclusion
AI Integration into day-to-day business is no longer a future nicety but a current requirement for organizations looking to remain competitive, nimble, and customer-focused. From enhancing effectiveness and data-driven decision-making to reducing expenses and providing hyper-personalization, AI is revolutionizing how businesses operate on all levels. But to unleash its potential, businesses need to integrate AI strategically, setting their sights on genuine pain points, laying quality foundations of data, and aligning their AI efforts with corporate objectives. Done correctly, AI does more than fuel business growth—it becomes a potent catalyst for innovation, resilience, and sustainable success.