After COVID-19, Artificial Intelligence (AI) became a useful ally in medicine. It helped doctors predict health complications, quickly diagnose diseases, and track the spread of infections. Hospitals were already exploring AI before the pandemic. But COVID-19 brought it forward, forcing them to use AI at a quicker rate to improve diagnosis, treatment, and the way they treat patients.
Now, the healthcare industry is experiencing a seismic change driven by AI technologies. No longer futuristic, AI is becoming the cornerstone, with 80% of hospitals already embracing it to enhance patient outcomes, operationalize activities, and reduce clinician workload (Forrester).
But with all the potential, there is a key question: What does it truly cost to deploy AI in healthcare?
This blog provides a full-on analysis of the cost, important considerations, and advantages of implementing AI in the healthcare sector, whether you’re a hospital executive, healthcare venture, or tech vendor looking into AI transformation.
Let’s explore what it involves—financially and strategically—to adopt AI in today’s healthcare.
How Much Does It Cost To Implement AI In Healthcare?
AI is transforming healthcare quickly, from automating diagnosis to simplifying medical documentation. But how much does it cost to deploy AI within a healthcare organization?
The short answer: Between $100,000 to $500,000+, depending on the type of AI model, data complexity, and depth of integration.
Here is a quick snapshot of the overall cost of AI in healthcare:
AI Model Type | Estimated Cost | Development Time | Use Case |
---|---|---|---|
Machine Learning (ML) Algorithms | $150K – $200K | 3 – 6 months | Predictive analytics, health risk scores, and data classification |
Neural Networks | $200K – $ 300 K+ | 6 – 9+ months | Complex diagnostics, treatment recommendations |
Generative AI / LLMs | $250K – $ 500 K+ | 6 – 12+ months | Medical chatbots, automated clinical notes |
Computer Vision Models | $180K – $ 400 K+ | 6 – 12 months | Radiology, dermatology, pathology imaging |
AI-Powered RPA | $100K – $250K | 3 – 6 months | Administrative automation – billing, scheduling, claims processing |
Key Factors To Consider While Implementing AI In Healthcare
Implementing AI in healthcare isn’t only a technical evolution — it’s a strategic shift. To thrive, you must take a look at the underlying factors influencing cost, performance, and long-term value. The following are the key factors you need to consider.
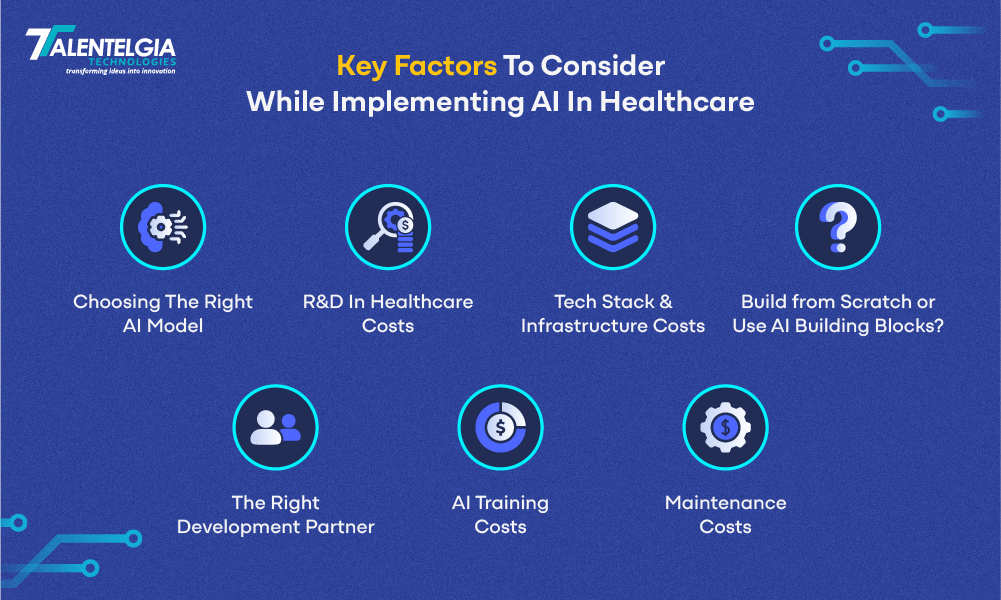
1. Choosing The Right AI Model
Not all AI models will be appropriate for your healthcare company. The price varies with the kind of AI integration your business requires. Here’s a straightforward division to give you an idea of what influences the price:
A. Standard ML Algorithms
These are great for fairly predictable applications such as patient segmentation, analysis of health trends, or warning signs of disease.
Development cost: Begins at $150K for a good MVP.
B. Neural Networks & Deep Learning
Used when dealing with large data sets or imprecise results — e.g., predicting low-incidence diseases or tailoring treatments.
Development cost: Tends to exceed $200K+, considering the changing, non-linear nature of such models.
C. Generative AI (LLMs)
Large language models such as GPT have the potential to transform physicians’ case documentation or communication with patients through smart chat.
Development cost: From $250K to $ 500 K+, particularly if adapted to clinical data.
D. Computer Vision Models
Computer vision is ideal for AI in medical imaging — from interpreting MRIs to detecting skin lesions.
Development cost: $180K to $ 400 K+, depending on training requirements and data quality.
2. R&D In Healthcare Costs
AI development goes way beyond just coding. It often involves exploring new ideas and solving complex problems, especially in healthcare, where accuracy and compliance are critical. That’s why a big part of your budget will go into research and development (R&D).
So, where does your R&D budget go?
- Hiring Specialized Experts
AI projects need more than just developers. You’ll need a team of specialists, such as:
- Data scientists crunch medical data and train their AI models.
- Machine Learning (ML) engineers develop and refine algorithms
- Clinical consultants familiar with healthcare regulations and patient safety
For example, if you’re creating an AI for disease diagnosis from X-rays, you’ll need radiology experts to label the images, data scientists to train the model, and ML engineers to make it run efficiently in your app. It’s a combined effort, and that raises R&D cost.
• Data Acquisition & Cleaning
To train an AI model, you need the correct type of data, and in healthcare, that is not readily available.
You require massive amounts of correct, labeled data, such as patient histories, imaging studies, lab tests, etc.
This data needs to be cleaned and prepared, i.e., to eliminate errors, fill in missing data, and get everything in the proper format.
Medical data is sensitive and HIPAA-protected, so you can’t simply download it off the web. You may need to purchase datasets, collaborate with hospitals, or collect your own, all of which cost time and money.
For example, to train an AI to identify skin cancer, you might need thousands of dermatologist-verified labeled images of various skin conditions.
• Experimentation & Model Tuning
Developing an AI model is not a one-off task. There are many tests and revisions before it can work correctly.
- Your initial copy of the AI will most likely not be flawless.
- You might need to experiment with various approaches, make revisions to the model, and continue optimizing it to achieve improved results.
This stage of the process may take weeks or even months. It’s similar to teaching a student — the AI must practice, get corrections, and receive feedback before it’s smart and reliable.
Example: If you’re creating an AI tool that can predict which patients will miss their doctor appointments, the initial version may not be accurate. But as you accumulate more data and tweak the model over time, it will refine itself.
3. Tech Stack & Infrastructure Costs
Developing scalable, future-proof healthcare AI software also entails:
- Selecting appropriate ML frameworks (such as TensorFlow, PyTorch)
- Comparing open-source (free and flexible) versus proprietary AI models (paid and controlled)
- Implementing solid DevOps and MLOps pipelines
- Meeting HIPAA, HL7, FHIR, or GDPR compliance standards
Using an architecture with care gets you out of technical debt — a prevalent trap that raises long-term costs and maintenance efforts.
4. Build from Scratch or Use AI Building Blocks?
If money is limited or you’re in the process of a rapid release, relying on pre-built AI building blocks is a wise choice. These frameworks limit development time and expenditures but still provide satisfactory performance, particularly for MVPs (Minimum Viable Products).
The following are some of the best choices to look into:
- Pre-trained ML models: Already trained on extensive collections of medical data and can perform everyday tasks such as disease prediction or medical image analysis with a few fine-tuning adjustments.
- Open-source NLP libraries: Ideal for developing healthcare chatbots or patient automation. They include medical vocabulary built in and can be fine-tuned accordingly.
- No-code or low-code AI platforms: Perfect if you don’t have an entire tech team. These platforms enable you to develop AI capabilities with easy drag-and-drop or with a very small amount of coding.
Tip: With these pre-existing solutions, costs can be decreased by 30–50%, so they are perfect for launching your product rapidly.
5. The Right Development Partner Can Halve Your AI Costs
Surprisingly, one of the largest contributors to the cost of AI in healthcare isn’t the technology — it’s how well (or badly) the execution is managed.
Selecting the appropriate development partner can be a huge game-changer, not only in terms of cost but also speed, compliance, and long-term ROI. A quality team doesn’t only develop your solution — they implement your vision with the proper strategy, expertise, and execution.
Here’s what to seek:
Established Experience in Healthcare AI:
Find a partner who already has experience in delivering AI-driven healthcare apps, is aware of medical data standards, and is knowledgeable in HIPAA or GDPR compliance. Experience decreases the learning curve and prevents expensive errors.
Cross-Functional Expertise:
AI development is not just about algorithms. It requires a full-stack effort. The best team should have:
- AI/ML Engineers to develop intelligent models.
- UI/UX Designers to design seamless, user-friendly interfaces.
- Backend Developers for handling infrastructure and integrations.
- QA Analysts for reliability.
- Compliance Experts to keep you on the right side of regulations
This balanced skill set results in quicker delivery and fewer blind spots.
6. AI Training Costs: A Hidden Technical Debt
One of the most significant change management challenges is training users. Implementing AI tools, especially in clinical settings, involves extensive training. Physicians, nurses, administrative personnel, and IT staff all need to be trained on using AI systems to maximize their benefits.
As Schell (AI adoption specialist) so aptly put it, “Training carries a huge technical debt.” It’s not merely about onboarding — it’s about creating digital confidence, establishing user trust in AI predictions, and minimizing friction in day-to-day life.
Training programs in healthcare AI generally consist of:
- Hands-on workshops for physicians and nurses
- Simulations for AI-aided diagnostics
- Workflow integration sessions for back-office staff
- Ongoing user support and documentation
These training sessions are not one-time events — they are an ongoing expense that needs to be included in the overall AI project budget.
Change management and training for AI in healthcare generally cost between 10% and 20% of the total AI project budget.
These figures vary based on:
- How big is your user base (number of physicians, nurses, staff)?
- Complexity of the AI tools involved
- Amount of workflow customization required
- How much refresher training and ongoing support is needed
Since training plays a key role in successful implementation and ROI, underestimating its cost can lead to delays, frustration, and project failure.
7. Maintenance Costs: Monitoring, Tuning & Compliance
Whether you’re using off-the-shelf Generative AI tools or custom-built machine learning models, every AI system requires consistent monitoring and updates.
Key components of AI maintenance in healthcare include:
- Model retraining with fresh, real-time data to ensure accuracy.
- Performance tuning to optimize outputs
- Governance and compliance checks to stay aligned with HIPAA and other regulations
- Cost control for token-based usage in pay-as-you-go GenAI tools
As AI technologies grow across various departments or buildings, maintenance will increase. Nevertheless, experts predict that improvements in AI efficiency, like DeepSeek’s lower computing power needs, will reduce long-term operating costs.
Quick Read: How To Develop Gen AI Solutions?
8. Hidden Costs
AI adoption in healthcare is usually accompanied by unseen costs that can undermine even the most well-planned budgets. Some of the most frequent surprises include:
- Steep data cleaning costs
- Sophisticated system integrations
- Extension of staff training requirements
Most organizations also neglect significant costs such as security audits, model tuning, and compliance checks.
According to a Deloitte report, 63% of healthcare AI initiatives have budgets that run 25% or more over because of these overlooked areas.
Pro Tip:
Prepare for emergencies, budget resources for long-term care, and match your technology to actual-world workflows to maintain your project timeline, particularly in deploying technologies like AI-powered patient outreach systems.
Benefits Of Implementing AI In Healthcare
Healthcare AI is more than a technology upgrade—it’s a game-changer that touches all levels of patient care and medical operations. From improving clinical accuracy to automating administrative workflows, AI is revolutionizing the way healthcare professionals work and patients are cared for. Let’s talk about some of the most notable benefits AI has to bring to the healthcare system:
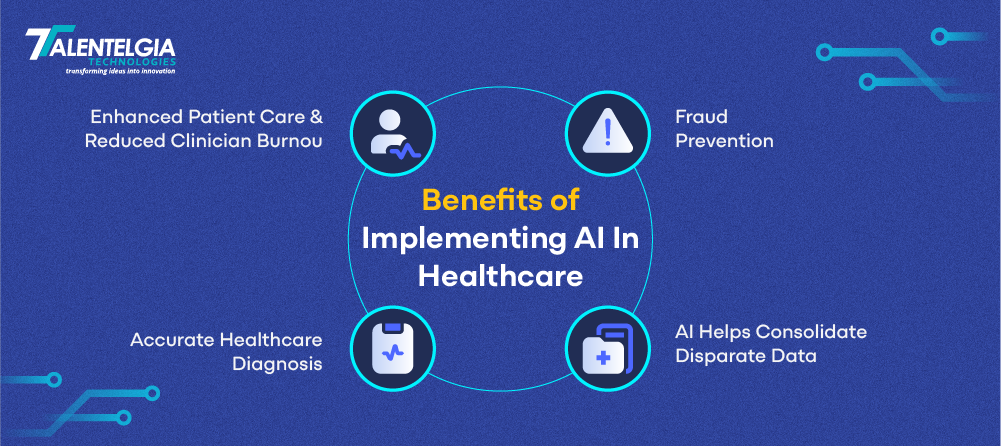
1. Enhanced Patient Care & Reduced Clinician Burnout
AI is changing the healthcare experience — not by substituting physicians, but by enabling them to spend more time with patients. For clinicians overwhelmed with workloads, AI tools such as medical scribes can document visit notes automatically, identify key information, and update electronic health records in real time.
Physicians can also use AI to:
- Write patient communication letters
- Condense patient histories to make better decisions
- Make data-driven, personalized treatment recommendations
By streamlining repetitive documentation and cognitive work, AI alleviates the workload and pressure on healthcare providers, preventing burnout and enhancing patient satisfaction. This harmonious blend of efficiency and empathy is where AI excels in contemporary care delivery.
2. Fraud Prevention
Healthcare fraud is a huge problem, costing the industry more than $380 billion each year and increasing premiums and patients’ out-of-pocket costs. AI-driven fraud detection systems can be an invaluable weapon in fighting this battle.
The systems can scan enormous amounts of claims data and identify warning signs such as:
- Billings for services never rendered
- Unbundling procedures to bill more
- Ordering tests that are unnecessary to make money
By catching suspicious patterns early, AI enables insurers to save on false claims, eliminate unnecessary payments, and, in the long run, lower healthcare costs for all, from providers to patients.
3. Accurate Healthcare Diagnosis
AI is starting to deliver on its promise of enhancing diagnostic accuracy and reducing healthcare costs. Harvard’s School of Public Health states that AI-based diagnostics can decrease treatment expenses by as much as 50% and increase health outcomes by 40%.
Some compelling applications are already on the horizon:
- Breast Cancer Risk Prediction: A University of Hawaii study employed deep learning to boost early detection. AI models are able to train on more than a million radiology images—many more than a radiologist will see in a lifetime—providing faster, scalable risk estimates.
- Hybrid Diagnostic Models: MIT researchers created an AI system that understands when to take a cue from human experts. For instance, in cardiomegaly detection on X-rays, a human-AI collaboration produced better results.
- Skin Cancer Detection: In a global study, a machine learning model that was trained on 100,000+ images performed better than 58 senior dermatologists at detecting skin cancer.
As such, technologies progress, AI can be an effective ally to doctors, providing quicker, more precise diagnoses and improved patient outcomes.
4. AI Helps Consolidate Disparate Data
Artificial intelligence is revolutionizing the collection, sharing, and utilization of healthcare information. By unifying disparate sets of data across devices and systems, AI allows clinicians to monitor patients’ health in real time and make quicker, evidence-based choices.
Consider the care of diabetes. According to the Centers for Disease Control & Prevention, more than 11.6% of Americans have diabetes. Wearable technology now tracks glucose levels in real-time and sends reports to care teams around the clock. AI reviews this data at scale to reveal patterns, tailor treatment, and enhance outcomes.
In addition to patient treatment, AI is transforming pharmaceutical drug safety and development. Firms such as Selta Square apply AI to optimize pharmacovigilance (PV)—a key, continuous process that identifies and avoids unwanted drug effects. Through PV automation, AI assists pharma companies in achieving safer drugs more quickly and cheaply.
In addition, artificial intelligence-driven simulations are reducing the reliance on in vivo testing of drugs. Algorithms can forecast toxicity, bioactivity, and even come up with new drug molecules, reducing R&D expenses while speeding up discovery.
From managing chronic disease to more secure, intelligent drug development, AI is enabling healthcare to shift from reactive to proactive.
Real-Life Examples Of AI In Healthcare
Numerous top healthcare organizations are already leveraging the potential of AI to revolutionize patient care and research. These practical uses demonstrate how AI is making a real impact—enhancing results, speeding up diagnoses, and simplifying intricate processes. Let’s take a closer look at some trailblazing examples:
1. Cleveland Clinic
At the Cleveland Clinic, AI is critical in the intensive care unit, as it facilitates early identification of patient deterioration. Their sophisticated AI algorithms keep monitoring real-time ICU data—vital signs, lab values, and other vital indicators, to identify faint warning signs that go unnoticed to human eyes.
This earlier intervention enables medical teams to treat complications more effectively, achieving better survival rates.
Moreover, the Cleveland Clinic uses natural language processing (NLP) to derive vital information from unstructured clinical documents, such as physician notes, discharge summaries, and patient histories. Through the automation of data extraction, AI speeds up clinical decisions and makes them more accurate, thereby optimizing workflows and enhancing patient care.
2. MD Anderson Cancer Center
MD Anderson Cancer Center unleashes the potential of AI to revolutionize cancer research and treatment by interpreting pathology slides and genomic information. The technique allows for customized treatment plans based on each patient’s individualized cancer profile.
With the incorporation of AI in its ontology processes, MD Anderson enhances diagnosis accuracy and treatment efficiency, resulting in improved patient outcomes and faster cancer research breakthroughs.
Classic microscopic examination is time-consuming and subject to human error, but deep learning from AI quickly and accurately identifies cancer cells in biopsy samples. This lets patients get quicker, more accurate diagnoses, enabling timely and focused care.
Conclusion
AI is rapidly reshaping healthcare with enhanced diagnostic precision, personalized therapy, improved efficiency, and optimized patient outcomes. Although implementation costs for AI will differ considerably in terms of complexity and integration, long-term value such as diminishing clinician burnout, reduction in fraud, and quicker research more than offsets the upfront expenditures, rendering AI a worthwhile strategic investment for health organizations in a quest to provide smarter, better, and more patient-oriented care. AI today is ushering in an improved, promising future of medicine.