Generative AI will be a $1.3 trillion market by 2032, Bloomberg Intelligence predicts—a sure indication of its explosive expansion and industry-altering potential. From automating creative work to driving smart assistants, GenAI is transforming how we engage with technology.
But with its ascendance comes a vital duty: making these AI systems equitable, unbiased, and fair. As generative AI models permeate decision-making, content generation, and practical applications, fairness is no longer desirable—it’s required.
So, what does fairness in GenAI mean? How can developers, researchers, and companies avoid discrimination, mitigate bias, and build systems that treat all users equally?
In this blog, we’ll unpack the meaning of fairness in generative AI, dive into its key principles, examine the challenges in achieving it, and outline best practices to guide ethical and inclusive AI development. Let’s get started:
What Is The Principle Of Fairness In Gen AI?
Fairness in generative AI refers to creating systems that generate unbiased outputs, provide equal treatment to all users. It aims to stop discrimination against people based on race, gender, age, or origin. This guideline is very important as generative AI has applications in hiring, healthcare, education, and finance.
For instance, if a recruitment tool is trained on biased data, it will tend to prefer male candidates over female candidates. Likewise, a healthcare chatbot can provide less accurate recommendations to underrepresented groups. Such problems can lead to real-world harm and erode trust in AI. To be fair, AI models should be trained on diverse data, tested for bias regularly, and made explainable.
Applications such as ChatGPT and DALL·E have come under fairness scrutiny, and updates have been implemented to enhance output equity. Fairness is not a single step in GenAI—it’s a process that must constantly be reevaluated and optimized. Through the inclusion of fairness at each development step, generative AI becomes more inclusive, ethical, and trustworthy for everyone.
Quick Read: How To Integrate ChatGPT In Your Website?
Major Components of Fairness In Generative AI
Fairness in generative AI isn’t a one-time action—it’s a structure based on several core principles. These principles assist in ensuring that AI systems treat all users equally, deliver balanced results, and facilitate ethical use at each step. The following are the fundamental building blocks of fairness in GenAI, presented in simple language.
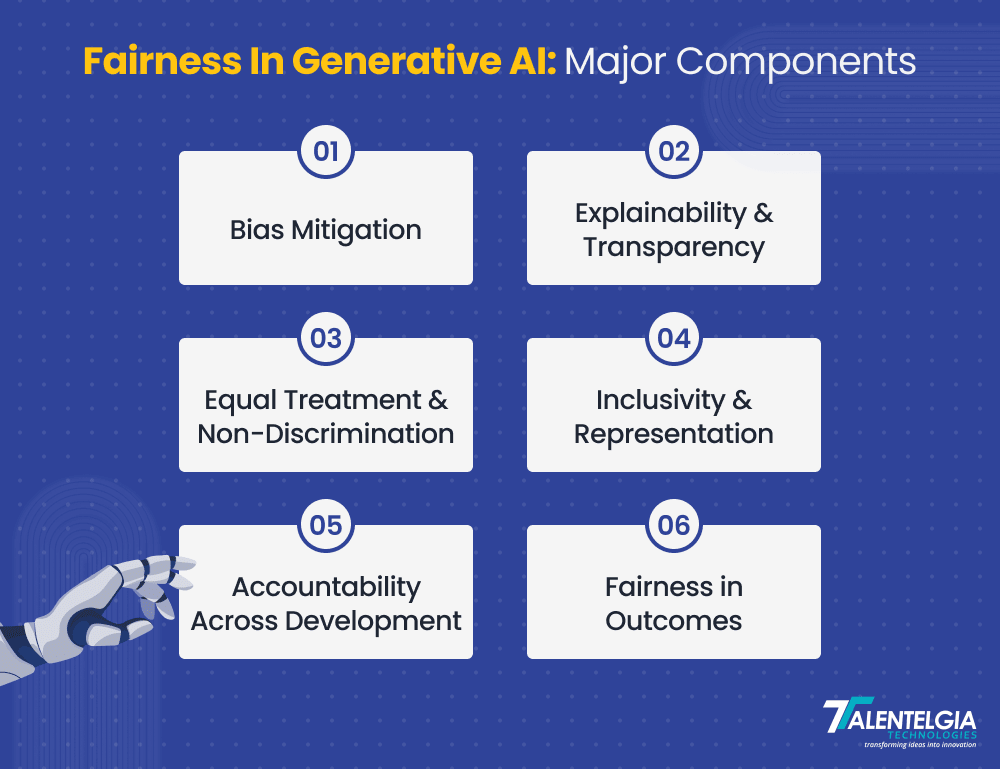
1. Bias Mitigation
Bias usually enters AI systems in the form of imbalanced or incomplete training data. For instance, if an AI system is trained predominantly on data from a single group, the system will do worse for others. This can produce disastrous, biased outputs.
To minimize bias, developers have to test models regularly and improve them with new, varied data. That’s known as iterative testing. It’s not a fix-and-forget-it solution—it’s a regular thing that makes the model better with time. The aim is to remove unjust outputs before they ever see users.
2. Explainability and Transparency
Humans are curious to know how AI comes to its conclusions, particularly when they impact employment, lending, or health. This is where transparency becomes relevant.
An open AI system prominently displays how it was created, what information it draws upon, and how it arrives at decisions. Explainability takes it a step further by providing users in plain language why a model returns a particular output. For instance, users ought to be informed why an AI rejected a loan or suggested a medical test.
This openness generates trust. If individuals know the way AI operates, they will be more likely to accept it and work with it confidently.
3. Equal Treatment and Non-Discrimination
Equal AI fairness isn’t just equal access—it’s equal results. AI systems must generate fair outcomes for men and women of all races, ages, and backgrounds.
To do so, developers can create performance standards for demographic groups. This guarantees no group is being discriminated against or left behind. Legal policies such as Europe’s GDPR also mandate businesses to create non-discriminatory systems, providing another level of responsibility.
4. Inclusivity and Representation
An AI system will only be equitable if it can grasp the individuals it serves. That requires training it on data that mirrors diversity in the real world, along with ethnicity, age, place, gender identity, and economic background.
Inclusive design also implies making AI tools usable by people with varying abilities. For instance, voice-driven systems must be accessible for people with speech disorders, and visual interfaces must accommodate screen readers.
Collaborating with cross-functional development teams throughout, such as ethicists, community leaders, and designers, also reveals unconscious bias and makes systems more inclusive.
5. Accountability Across Development
Accountability translates to organizations fully owning up to their AI systems, from collecting data to deployment. That means developing internal ethics rules and independent audits to detect problems early.
Consistent monitoring is a must. Developers actively need to gather feedback and correct any unfair trends in real-time. It is simpler to be fair as the AI develops when accountability is integrated into each step.
6. Fairness in Outcomes
Being fair on paper isn’t enough for an AI system—it needs to be fair in real life, too. That entails testing how the AI acts in real situations and making sure its outputs don’t hurt any particular group.
Take hiring or medicine, for instance. Biased outcomes there can have dire repercussions. Developers need to employ fairness metrics to see how the AI impacts various groups and tweak it accordingly.
Fair outcomes indicate that the system performs as designed and promotes ethical objectives. Ongoing assessment serves to maintain the technology consistent with user requirements and social norms.
Quick Read: Generative AI Vs Traditional AI
Challenges In Ensuring Fairness In Generative AI
While it’s critical to incorporate fairness into generative AI, it’s not easy by any means. Engineers encounter a variety of technical, social, and philosophical challenges when attempting to mitigate bias and achieve fair outcomes. The next step in creating more responsible and inclusive AI systems is understanding these challenges.
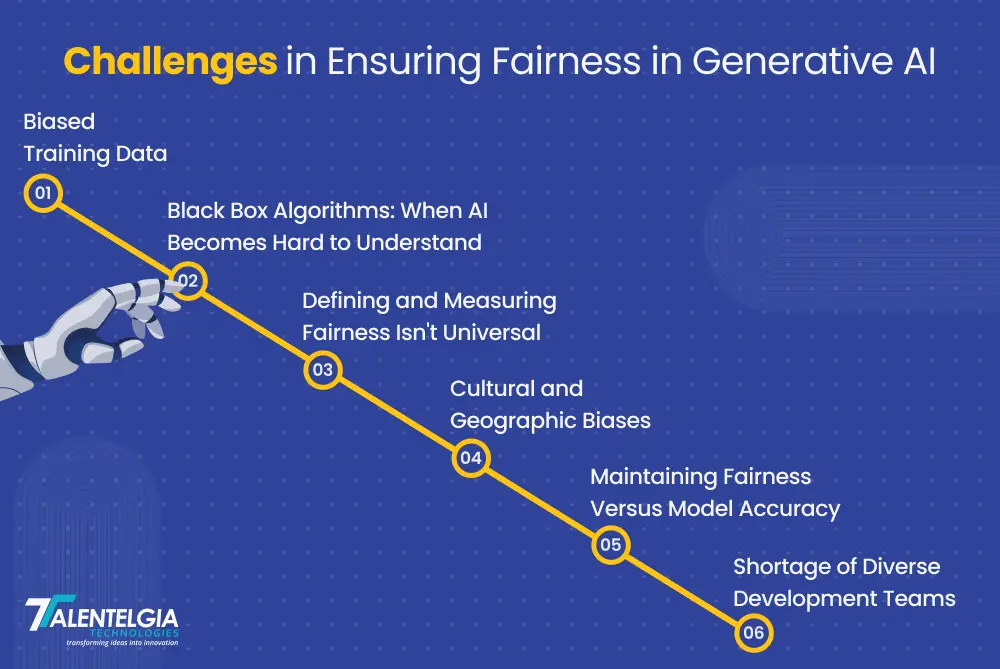
Some of the most important challenges are as follows:
1. Biased Training Data
Problem: Most generative AI models are developed using large amounts of data collected from the web, news sources, books, and historical writings. Unfortunately, these sources do have built-in biases, such as gender biases, racial biases, or economic biases.
Why does it matter?
If your training dataset leans toward one group or demographic, the AI will reproduce that bias, producing unbalanced, unjust, or even harmful results. Once such biases have been ingrained in the model, de-biasing them is a huge technical challenge.
For example, in 2022, Apple faced allegations that the oxygen sensor in its Apple Watch was racially biased, a consequence of biased data influencing the AI’s performance. Likewise, Twitter’s (now X) default image-cropping AI was accused of being pro-male and pro-light-skinned, further establishing the influence of biased training data. These instances illustrate how pervasive biases can taint the fairness of AI systems.
2. Black Box Algorithms: When AI Becomes Hard to Understand
Problem: Most generative AI models, particularly those based on deep learning, are black boxes—you can look at what’s put in and what’s taken out, but not what is done in the middle.
Why is it challenging?
It becomes difficult to recognize where the bias is coming from or how it’s being decided. Without a view, addressing fairness is all about guessing.
3. Defining and Measuring Fairness Isn’t Universal
Fairness isn’t universal. What one group perceives as fair may be discriminatory to another.
Why it’s difficult?
There is no one-size-fits-all fairness metric. Creating effective AI fairness benchmarks across demographics, languages, and cultures continues to be a challenge.
4. Cultural and Geographic Biases
Global AI, Local Blind Spots: Generative AI learned primarily from data for a single region (such as North America or Europe) could be ignorant of the sensibilities, values, or dialects of other regions of the world.
The result?
Outputs will inadvertently offend or exclude individuals from underrepresented cultures. That is why the diversity of datasets globally is necessary.
5. Maintaining Fairness Versus Model Accuracy
The trade-off in performance: Enhancing fairness might decrease a model’s performance on some tasks from time to time. For example, removing biases in data might decrease usable information, affecting output quality.
The Dilemma:
Do you compromise on accuracy to achieve equity? Finding equilibrium between fairness and efficiency is an ongoing balancing act for AI teams.
6. Shortage of Diverse Development Teams
Why diversity is important in AI development: When AI teams are not representative of diverse backgrounds, they’re more likely to overlook blind spots, such as biased wording or exclusionary outputs.
The solution:
Assembling inclusive, interdisciplinary AI teams allows for hidden issues to be caught early in the design process, enhancing both equity and relevance.
Also Read: Generative AI Challenges & Their Solutions
Fairness In Generative AI: Best Practices
As generative AI continues to shape decisions in areas like healthcare, hiring, finance, and education, ensuring fairness isn’t just a nice-to-have—it’s a must. Biased algorithms can reinforce discrimination, amplify inequality, and erode trust. To build AI systems that are ethical, inclusive, and accountable, organizations must follow a structured approach grounded in fairness.
Here are four proven best practices to help achieve that:
1. Leverage Diverse and Representative Datasets
The basis of an unbiased AI model is the training data. If your datasets are biased towards a single demographic or cultural perspective, your AI will probably carry that bias forward in its output.
To fix this:
- Utilize inclusive datasets that cover various races, genders, socio-economic classes, and cultures.
- Regularly audit your data to find underrepresented groups or hidden patterns.
- Implement oversampling methods to increase visibility for minority groups.
- Eliminate unjust correlations that may generate discriminatory outputs.
By deliberately hand-curating your datasets with equity in mind, you minimize the risk of entrenching stereotypes and enable the model to learn from a broader spectrum of human experience.
2. Incorporate Fairness-Aware Algorithms and Techniques
Fair AI does not occur spontaneously—it takes conscious intervention during model training. Now, there are specialized algorithms that can help reduce bias and foster equitable outcomes.
Well-known approaches are:
- Reweighting datasets to avoid overrepresentation of majority groups.
- Forcing the model to unlearn toxic correlations with adversarial debiasing.
- Imposing fairness constraints on directing the model towards fair decision-making.
Regular monitoring using fairness measures—such as demographic parity, equal opportunity, or disparate impact—ensures these techniques are operating effectively. Testing and tuning these algorithms in both development and deployment phases is critical.
3. Encourage Transparency and Explainability
Transparency is a significant stumbling block to fairness in AI. If users cannot see how decisions are being made, it is impossible to recognize or correct unfairness.
To enhance transparency:
- Write down your data sources, model selections, and decision logic.
- Employ explainability tools such as SHAP, LIME, or attention heatmaps to graphically show how the model comes to particular outputs.
- Open your systems to audit by internal teams or third-party experts.
These initiatives construct user trust, particularly in sensitive fields such as health, employment, or money, where biased choices can have severe ramifications.
4. Engage Multidisciplinary and Inclusive Teams
AI fairness must never be a task left for developers alone. Developing inclusive technology involves consultation from individuals with varying expertise, experience, and values.
Best practices are:
- Consulting ethicists, sociologists, legal experts, and field experts.
- Involving marginalized community voices in product design and testing processes.
- Implementing various review boards to analyze fairness issues from varied perspectives.
Having mixed groups consisting of technical and non-technical members is more likely to discover underlying biases, challenge assumptions, and come up with socially and culturally responsive solutions.
Real World Example Of Fairness In Generative AI: Adobe Firefly
When Adobe introduced its Firefly generative AI tools, it took a clear and confident stance on transparency, something that sets it apart from many other AI platforms. Unlike other tools such as OpenAI’s DALL·E, Adobe publicly disclosed the sources of the images used to train Firefly. The company emphasized that all training data comes either from Adobe’s licensed image libraries or from public domain content. This amount of transparency enables users to believe that Firefly isn’t constructed on copyrighted content without authorization, providing creators with peace of mind and authority over the way they use AI-generated imagery.
Conclusion
Generative AI can reshape industries, enable creativity, and redefine how we engage with technology. That power does come with great responsibility. When these models make decisions in healthcare, hiring, finance, and beyond, making them fair isn't an ethical afterthought—it's a necessary foundational element.
Generative AI fairness isn't a checkbox to check or a once-and-done solution—it's an ongoing process that requires purposeful effort, inclusive thinking, and collaborative problem-solving. From diverse training data and explainability to bias prevention and inclusive development teams, each step of the AI pipeline needs to be purposefully designed to maintain equity.
By integrating fairness as a fundamental value—instead of an addendum—we not only develop better tech, but systems that mirror and benefit the diversity of the real world. Generative AI can only achieve its full potential then: not as a tool for innovation, but as a driver of positive, inclusive change.